AI in B2B Lead Scoring: The Complete Guide for Success
If you still rely on manual lead scoring in your sales process, chances are you rely heavily on human assumptions and waste time on unqualified prospects rather than focusing on those who are ready to buy.Â
However, the rise of AI lead scoring makes it easier to identify key signals that enable sales teams to score and prioritize leads. Backed by advanced technologies like machine learning and predictive analytics, sales teams can score leads faster and tailor their efforts to those who are most likely to convert.Â
This article further explores the concept of AI in B2B lead scoring with certain key points like:
- How AI transforms lead scoring and boosts B2B marketing efficiency
- How to use AI lead scoring to enhance your B2B sales strategy
- Best practices for implementing AI-driven lead scoring in B2B sales.
Whatâs AI Lead Scoring?
AI lead scoring is the application of artificial intelligence and machine learning algorithms to analyze prospectsâ data and rank leads based on their conversion potential. AI lead scoring model eliminates manual efforts, which are time-consuming, and allows sales teams to prioritize their efforts on leads that are most likely to convert.Â
Letâs say you have 1,000 prospectsâ data to analyze and sort based on their recent visit to your website. AI lead scoring software can immediately capture the signals and assign a score based on the leadâs interaction with your site. For instance, leads that visit the pricing page may be assigned a higher score than leads that visit the homepage.Â
This process happens in real time, allowing you to identify and prioritize your most promising leads, greatly increasing the chances of converting them into real sales.Â
Traditional vs. AI Lead Scoring in B2B Sales
Traditional lead scoring is like throwing darts in the dark. Salespeople spend hours surfing various data sources such as job titles, industries, company sizes, website visits, or form submissions to analyze and qualify leads based on their attributes and behaviors. Theyâd use their assumptions or best judgment to determine the leads they should prioritize based on how closely they match the companyâs ideal customer profile.Â
The result of this manual analysis was pretty simple: Salespeople categorize their leads in a binary formââhot or cold,â âgood or bad,â or âqualified or unqualified.â Leads are tagged âqualifiedâ if they match pre-defined criteria and âunqualifiedâ if they donât. This approach resulted in an inaccurate lead scoring model, enabling the sales team to potentially overlook valuable leads.
On the contrary, AI-powered lead scoring develops a more holistic approach to scoring and qualifying leads. Analyzing data from various sources is one of its core fortes, as this process identifies behaviors, characteristics, and patterns that show the highest likelihood of conversions. This results in an accurate scoring process that helps sales teams prioritize the most promising leads, boosting their lead-generation efforts.Â
Also, itâs essential to note that the manual lead-qualifying process is time-consuming and prone to human errors and biases. Additionally, salespeople who use the traditional lead scoring model find it challenging to update scores based on recent data and miss out on important details. These dynamics prevent them from adapting to lead behavior changes over time, thus affecting their scoring model as new interactions occur.Â
However, AI B2B lead scoring tools qualify leads based on the data from different sources, allowing sales teams to identify leads who are more likely to convert. Unlike the manual approach, they regularly update the data in your CRM to ensure an accurate scoring model that aligns with your current sales efforts.Â
How to Use AI to Create a More Accurate Lead Scoring ModelÂ
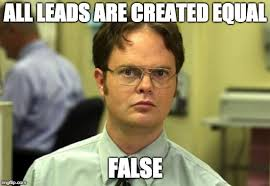
â
AI in B2B sales performs various functions across different sales processes. In the case of lead scoring, AI collects and analyzes data from different sources to eliminate the biases associated with manual methods and improve the accuracy of lead scoring. Manual scoring is often influenced by the sales repâs decision, which may be inaccurate and result in missed opportunities. AI removes this subjectivity by analyzing various data sources and utilizing their insights to score and qualify leads.Â
When asked about how he uses AI to score leads, Dujam Dunato, GTM Engineer at ColdIQ, says, âI use AI to score leads primarily by extracting and analyzing information from leadsâ websites, LinkedIn profiles, and other public data sources, then matching that enriched data to a predefined Ideal Customer Profile (ICP). For example, I might use AI tools, such as Perplexity, to summarize a companyâs product offerings and market positioning, and then compare those findings to specific criteria like industry focus, company size, revenue range, or technical stack. If the lead matches enough high-value criteria, it receives a higher score.â
With defined criteria and enriched data, AI can help human sales reps identify hidden patterns, tweak criteria, and identify new factors to improve targeting and refine their ideal customer profile. As more data flows through the system, a continuous cycle of optimization keeps the lead scoring model highly accurate to provide actionable results.
Related: 8 Best AI Lead Generation Tools in 2025
Key Data Points AI Uses to Improve Lead Scoring
Unlike traditional methods that use limited data points, AI leverages data points from various sources to accurately score and qualify leads. These data points include:
1. Behavioral Data (Website visits, email engagement, downloads, etc.)
Behavioral data showcases a leadâs interaction with your brand across various touchpoints. This includes actions like website visits, social media engagement, email clicks, and content downloads. These interactions enable AI to gauge the level of interest a lead has in your business offerings.Â
For instance, a lead who spends time on high-value pages like pricing is interested in your products and is more likely to buy those products or services. This action allows the lead scoring model to score the lead higher than others who didnât show interest.Â
2. Firmographic Data (Company size, industry, revenue, etc.)
Firmographic data refers to the information related to a company. This includes the company size, industry, revenue, headcounts, location, and the leadâs role. AI leverages this data to determine whether a lead aligns with your ideal customer profile (ICP).Â
For instance, if your B2B solution is for companies with at least $10 million in annual recurring revenue, sales teams can leverage AI to generate high-intent leads in this area. This allows your sales teams to focus on the high-value leads that are most likely to convert.Â
3. Technographic Data (Tools and Software Used By Prospects)
Technographic data are the tools and technologies used by a company in running its business activities. This can be auto-dialers, AI note-takers, CRMs, CMS, AI, blockchain, and more. AI can utilize this data to determine your leadâs digital landscape and gauge whether it fits your ICP. For instance, if you believe a company currently needs your B2B solution but doesnât have it in its tech stack, you can seize this opportunity to bridge the gap.Â
4. Intent Data (Search Behavior, Social Media Signals, Ad Interactions)
Intent data or sales triggers are powerful indicators that show a leadâs readiness to buy a product or service. While these signals are powerful, leveraging them at the right moment is the key to unlocking potential sales opportunities.Â
Florin Tatulea, Head of Sales Development at Common Room, says, âIntent data is powerful but only useful if you reach out immediately. In most cases, it's not useful if you reach out 2 weeks later and this is where a lot of teams get screwed.âÂ
To avoid this scenario, AI can help to find this data faster and connect you with leads with high purchase intent. With these data-driven insights, sales teams can reach out at the right moment, increasing the chances of conversion.Â
Related: 11 Best B2B Sales Prospecting Tools in 2025
How to Use AI Lead Scoring to Enhance Your B2B Sales Strategy
Here are a few ways to use AI lead scoring to enhance your B2B sales strategy.Â
1. AI-driven prioritization of high-value leads
A traditional lead scoring model wastes the sales repsâ time, especially when scoring and qualifying leads. Linda Lian, CEO and Co-founder of Common Room, says, âReps spend a big slice of their time struggling to understand which prospects are worth their time and piece together context so they can personalize outreach.â
However, AI identifies high-value accounts that are most likely to convert, allowing your sales teams to tailor messages and prioritize their efforts on high-value leads. This targeted approach will not only shorten the sales cycle but also improve conversion rates, as teams will only spend time with leads who are genuinely interested in purchasing your products or services.Â
2. Eliminates Subjectivity based on Guesswork
Traditional lead-scoring models often rely on the subjective opinions of sales professionals. With limited data and static criteria, they rely on their assumptions or make guesses on leads, allowing potential customers to fall through the cracks.Â
However, AI updates data spontaneously to score leads, resulting in a more accurate process that delivers results. This process further automates repetitive tasks and removes human biases from the process, ensuring no valuable leads are lost. Â
3. Constant Segmentation and Lead Routing
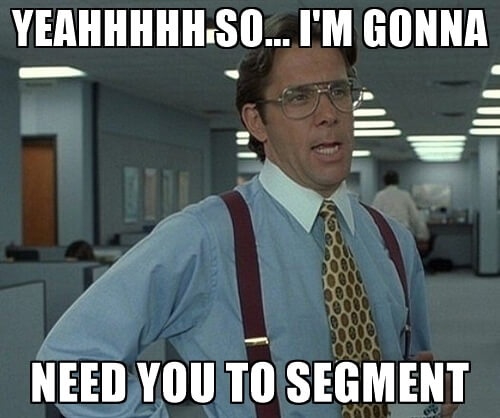
â
With the sales team often overwhelmed by a large volume of leads, AI lead scoring assigns scores and categorizes leads for easy sorting. It classifies these leads into tier A, B, and C groups or hot and cold leads.Â
These segmented accounts are then routed to sales reps who nurture leads with personalized outreach. With this consistency, sales reps learn how to handle leads effectively and increase the chances of conversions.Â
4. Optimizes Marketing Processes to Find High-Value Leads
With AI-powered lead scoring models in place, the marketing and sales teams can double down on their activities to drive efficiency. Rather than spreading resources on unqualified leads, marketing teams can channel those resources to create content, distribute it on the channels, and optimize campaigns to perform well.Â
On the other hand, sales reps can utilize training data to accelerate deal closure with qualified leads. This improves customer interactions and becomes an optimized engine that drives revenue growth for businesses.Â
Challenges of AI-Driven Lead Scoring
Like any technology, AI-driven lead-scoring solutions have their limitations. Here are some of the challenges salespeople may face when using the tool.
1. Data Quality

â
AI models often rely on the data from which they are fed. When that data is outdated or inaccurate, the AI model may fail to deliver accurate predictions and actionable insights. Hence, businesses should obtain data from reputable platforms to avoid being misled.Â
In an interview, Dujam Dunato, GTM Engineer at ColdIQ, says, âI usually supplement with data from LinkedIn or domain-level analyses, which can be more expensive but provide a richer perspective on the lead.â Additionally, businesses should perform data cleaning practices such as updating databases, verifying data accuracy, and removing duplicates to have a solid foundation for their sales process.Â
2. Wrong Prompting
When you use the wrong prompt with an AI model, chances are it can generate inaccurate or irrelevant results. For instance, if the prompt isnât specific enough, it can include leads that vaguely match your ICP. As a result, salespeople should craft highly-specific prompts, addressing what they want and donât want.Â
Dujam Dunato, GTM Engineer at ColdIQ, says, âThe AI will only be as good as the information and instructions it receives. So, I address this by refining my prompts to be more specific, training the AI to look for exact keywords or signals, and always performing a brief human review on edge cases to catch mistakes. By iterating through small trials and continuously refining criteria, you can get a solution that is both highly accurate in scoring leads and efficient at generating the right targets for your pipeline.â
3. Over-reliance on AI
AI models arenât totally perfect. SDRs who depend on their judgment or assessment could end up with an unreliable scoring model. For instance, if the system fails to tweak pre-defined criteria based on new data, that could result in wrong predictions that would affect your entire sales process. As a result, your lead-scoring solution should have human oversight where your sales teams make the final call to avoid fatal errors.Â
The Impact of AI-Driven Lead Scoring: Examples
Letâs explore two real case studies of how AI-driven lead scoring can transform sales efforts, driving better outcomes for businesses.Â
Example 1: Grammarly Increased Its Upgraded Planâs Conversions by 80%
Grammarly, a renowned AI writing partner, faced challenges gathering qualified leads to advance its sales efforts. The firmâs marketing team would manually build email lists based on user open rates or perceived interests in upgraded plans. While they would send more than 400 marketing qualified leads (MQLs) each month, the list often contained spam bots or accounts that werenât ready to convert, wasting the sales teamâs time.Â
However, Salesforceâs AI lead scoring features, such as Account Engagement and Einstein Account Insights, would help to predict and score leads based on their interactions with the firm. This action enabled Grammarly to prioritize quality leads over quantity. Marketing leads now send about 200 high-quality MQLs monthly, resulting in 30% conversions and an 80% increase in upgraded plans.
Example 2: HES FinTech Witnessed a 40% Increase in Loan ApprovalÂ
HES FinTechâs sales teams approached lead qualification with manual efforts. They engage in activities such as searching for additional information about leads or conducting demos, wasting their time and resources.Â
However, HES FinTech integrated machine learning into its lead scoring process, analyzing years of lead data in its CRM to build a predictive model. This approach resulted in a 40% increase in weekly approvals while reducing the time spent on lead qualification and driving the efficiency of their sales team.
Best Practices for Implementing AI-Driven Lead Scoring in B2B Sales
Adopting an AI-powered lead scoring model can transform your sales process. However, harnessing the full potential of the solution requires implementing some best practices to boost your sales efforts. This section explores some best practices to implement an AI-driven lead-scoring model for your business.Â
1. Identify Your Target Audience
Since many AI-driven lead scoring solutions are tailored towards specific audiences, itâs ideal to find one that fits your buyer persona. For instance, an AI-powered platform that offers intent signals will find relevant signals that show a prospectâs level of interest in a product or service.Â
All you need to do is ensure the platform takes all the data points into account to enable effective lead scoring. To achieve this, sales teams may need to define their ideal customer profile (ICP) and consider relevant factors like employees, industries, job titles, technologies used, and more. This helps to set up the criteria for lead qualification and ensure the AI lead scoring model qualifies the prospects appropriately.Â
2. Start with a Clean and Robust Data
An AI-driven solution is equally as good as the quality of data received. This serves as the foundation of any lead-scoring process. Having high-quality, comprehensive data helps analyze buying signals effectively to qualify leads.Â
For instance, if youâre targeting a company, you may need to include data points such as job titles, company size, industry, annual revenue, technologies used, and headcount. On the other hand, if youâre targeting individuals, you may want to consider data points such as demographics, contact information, and online behavior.
In any of these cases, ensure that the data is clean and up-to-date. Ensure theyâre free from inconsistencies or duplicates, as inaccurate data can mislead the lead scoring model. Perform audits regularly to maintain quality data. Remember that even the most capable lead-scoring software canât work effectively without a clean and robust dataset.Â
3. Monitor and Optimize Performance
While the AI lead scoring model improves over time, itâs essential to continuously monitor the process. Track key metrics such as sales cycle length or lead conversion rates to gauge their effectiveness and enhance accuracy. If you notice that the lead scores donât align with the outcome, you may want to tweak the AI model.Â
Ensure to regularly review the data inputs, scoring criteria, and predictive models to stay on track. This ensures that your scoring model aligns with your businessâs needs and fits your business goals.Â
4. Adapt to Market Changes
Since the B2B sales landscape is always evolving, chances are your leadsâ behavior is also adjusting to fit the market. As a result, itâs essential to adapt your AI model to these changes. Update your model to fit the latest trends, adapt to your customersâ preferences, and align with changes in industry development. Stay open to new data sources and scoring variables to improve your scoring model and optimize for future opportunities.Â
Partner with ColdIQ to Transform Your B2B Sales Strategy with AI Lead Scoring
Traditional lead-scoring methods waste time and resources, distracting sales teams with unqualified leads that frustrate their efforts. However, AI-powered lead scoring resolves this issue by analyzing vast amounts of data to identify the most qualified prospects. With a focus on behavioral, firmographic, technographic, and intent signals, it constantly assigns lead scores to leads with high conversion potential. This enables the sales team to prioritize their efforts and achieve high conversion rates.Â
Ready to transform your lead scoring process with AI? Partner with ColdIQ to implement AI sales tools in your lead management process and boost sales performance. Book a demo today!
AI Lead Scoring â Frequently Asked Questions (FAQs)
1. What is AI-powered lead scoring, and how does it work?
AI-powered lead scoring refers to the use of an AI tool to analyze a prospectâs data and evaluate potential customers based on their likelihood to convert, automating lead prioritization and driving sales efficiency.
2. What data points does AI use for B2B lead scoring?
AI uses data points such as behavioral, firmographic, technographic, and intent data to improve B2B lead scoring, focusing on various sources such as CRM, website interactions, social media platforms, email campaigns, and marketing automation.Â
3. How does AI-driven lead scoring improve sales and marketing alignment?
AI-driven lead scoring improves sales and marketing alignment by identifying high-quality leads, providing actionable insights, and streamlining workflows, resulting in increased collaborations and improved conversion rates.Â
4. What are the best AI software solutions for B2B lead scoring?Â
Sales teams can consider AI software solutions such as HubSpot, Salesforce, Zoho CRM, Einstein, and Factors.ai for B2B lead scoring.Â
5. How do I implement AI-based lead scoring in my sales process?Â
To implement AI-based lead scoring, start by defining your ideal customer profile, collecting and cleaning your historical data, choosing and training a suitable AI model, and monitoring and refining performance for optimal use.Â
â